Jobs
Bonus Episode: Lessons From Jobs in the Age of AI
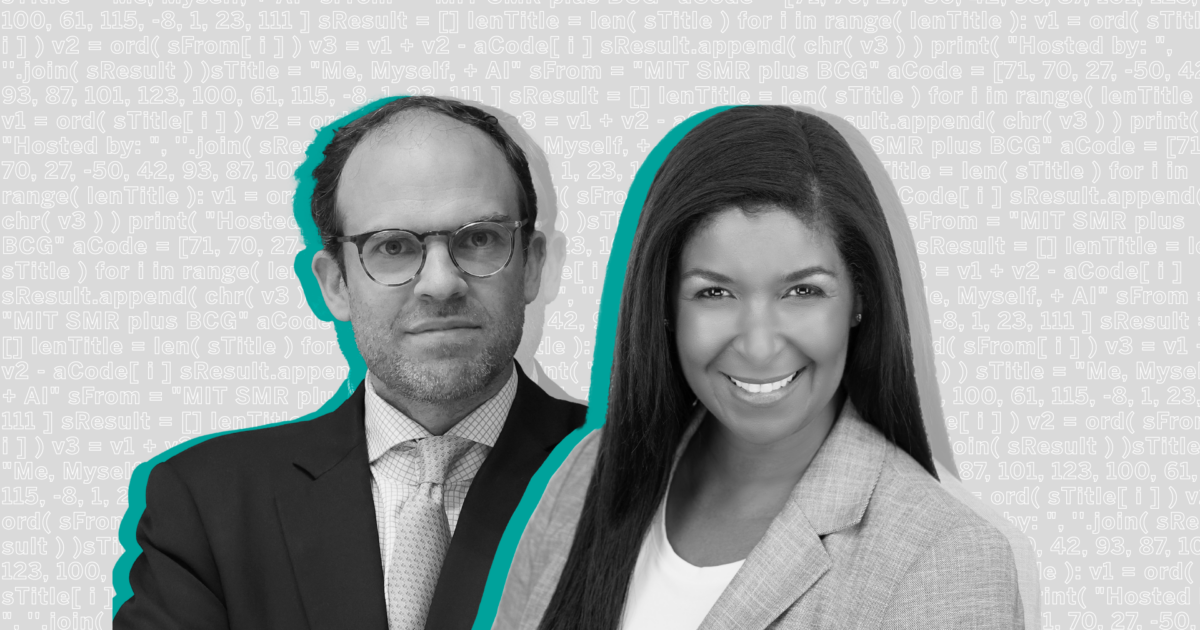
Topics
Artificial Intelligence and Business Strategy
The Artificial Intelligence and Business Strategy initiative explores the growing use of artificial intelligence in the business landscape. The exploration looks specifically at how AI is affecting the development and execution of strategy in organizations.
More in this series
On Sept. 4, 2024, Me, Myself, and AI host Sam Ransbotham moderated a panel discussion at a Georgetown University/World Bank event, Jobs in the Age of AI. Afterward, he interviewed keynote speaker Carl Benedikt Frey, Dieter Schwarz Associate Professor of AI and Work at the Oxford Internet Institute, and panelist Karin Kimbrough, LinkedIn’s chief economist. In this bonus episode recorded during this discussion, hear from Frey and Kimbrough about how artificial intelligence is impacting workers, labor trends, and the economy.
For further information:
Subscribe to Me, Myself, and AI on Apple Podcasts or Spotify.
Transcript
Sam Ransbotham: Hi, listeners. I’m here with Tim DeStefano, associate professor of research at Georgetown University’s McDonough School of Business. We are here at the World Bank/Georgetown event, Jobs in the Age of AI, which is part of the AI in Action series that Tim along with Jon Timmis have put together. Tim, can you give our listeners a brief overview of the event and how it came to be?
Tim DeStefano: Absolutely, Sam. Jon and I initially put this AI in Action conference series together with the objective of creating a forum where industry experts, policy makers, and academics — the key leaders of AI — come together and share knowledge about policy and artificial intelligence.
The purpose of today’s conference is to provide real-time information on the extent to which artificial intelligence is going to affect existing jobs as well as jobs in the future. The way in which the conference is organized is that we’ll start with academics who are going to share real-time data and academic research on the extent to which artificial intelligence is beginning to affect jobs. And then we’ll follow up with industry experts who will share examples of how AI is actually being implemented within firms and how it’s beginning to affect employment.
Sam Ransbotham: Sounds great. We’ve got two speakers from the event who are going to join us for our podcast episode today. So let’s get started with the first one.
Our first guest is Carl Frey. He’s from the University of Oxford and the keynote speaker at the Jobs in the Age of AI event at Georgetown and the World Bank. Picking up on his keynote, I thought I’d ask a few questions. First, you have a very influential paper about how skills and jobs are going to change in our coming future. At the time you were thinking creativity, social intelligence, and perception were going to be the big key trends in the future. What’s changed about your thinking?
Carl Benedikt Frey: Back in 2013, as you mentioned, we outlined three key bottlenecks to automation. One of them was complex social interactions. The state-of-the-art chatbots at the time are quite well exemplified by Eugene Goostman, which was a chatbot that tried to mimic human capabilities and pretended to be a 13-year-old [boy from Odessa, Ukraine] speaking English as his second language, and essentially [fooled] a lot of people in Loebner Prize competitions that it was actually a person. The chatbots that we have today are obviously much more capable, right? There’s no question that in the virtual space, at least, we’ve seen a lot of progress when it comes to automation. But I think what’s very likely is that as you see these technologies improve, it’s going to increase the value of in-person communications.
Think of it this way: If AI writes your love letters and everybody else’s, the first date becomes more important. As a company, if everybody is selling their product using AI, how do you distinguish yourself in such a marketplace? Well, it’s going to be through in-person communications. I think that bottleneck still holds at least in part. A second bottleneck has to do with creativity. Obviously, creativity is hard because we struggle to define it in the first place, but it essentially has something to do with coming up with novel ideas and artifacts that somehow make sense, that have some commercial or symbolic value.
I think it’s still the case that when it comes to frontier capabilities, we are still quite far off from automating those away when it comes to creativity.
Sam Ransbotham: So even if we have Cyrano de Bergerac writing our love letters for us, an automated AI version of that, still the first date is important. What you’re speaking to is the idea that some things have become scarce and some things have become not scarce.
Carl Benedikt Frey: Exactly.
Sam Ransbotham: And that’s changing on us.
Carl Benedikt Frey: Yes.
Sam Ransbotham: What’s going to be scarce coming up?
Carl Benedikt Frey: I do think that everything that is in person is more likely to become scarce to begin with, for example. And I do think that these algorithms are very good at rehashing existing concepts and, to some degree, also prediction. But a lot of the things that we do are more than just extrapolating from past patterns, right? If you would have trained an algorithm in 1900 to predict [whether] human flight could be possible, you would have essentially had an algorithm playing through a lot of failed experiments.
You might have looked to data on birds, which suggested that it’s possible to fly in the first instance. But even if you look at that data, you would have found that birds that weigh more than 50 pounds tend not to fly. And below that they rise with a lot of difficulty.
Humans do more than that kind of extrapolation. We build mental models of the world, we build theories, and those theories allow us, essentially, to come up with inventive ideas of how we can restructure our environment to make new things happen.
If you extrapolate just from past patterns of productivity growth, what I will suggest is that the economy is likely to be stagnant for some time. And if we go back and look at data over the past 200 years, we’re actually seeing that the growth rates have varied quite significantly. [After] a very significant productivity growth — 1920 to 1970 — that then tapers off. We don’t see a sort of brief rebound [between] 1995 to 2004, and then we see a flatlining again.
So if you extrapolate from any given point in time there, you’re quite likely to be wrong. What we learned from that is that we’re better off maybe looking at what’s actually happening in technology. And trying to understand how those technologies might reshape the economy. But obviously that’s a guessing game, too.
Sam Ransbotham: That’s the difficult thing about the future. It’s hard to know what’s next here. I think if we ask most people on the street about artificial intelligence, their mental model would quickly go to a robot probably influenced by Hollywood and other things. I think your example from the keynote was the gas lighter or the washing machine. That doesn’t seem to be what happens.
Carl Benedikt Frey: No. Most automation actually happens through simplification. We didn’t build robots by performing the motions of handwashing and then walking up to the house to hang the clothes to dry. We did that by inventing the electric washing machine.
In most contexts, automation happens through some sort of simplification, and that’s where a lot of the creativity comes in. If all you do to try to understand the future potential of automation is look at the composition of tasks in a given job and say that, “This is automatable, but that is not automatable,” you’re very likely to miss a lot. You would conclude, for example, that to drive a car you need finger dexterity because you need to hold the steering wheel.
But nobody is going to hold the steering wheel when driverless cars arrive. I think it’s quite easy to miss a lot of the things that are, in fact, quite likely to be automated using that approach.
Sam Ransbotham: One of the other points you made [that] I thought was interesting was how much of this is concentrated in the English-speaking world, and most of the jobs in the service industry are in the English-speaking world. But that doesn’t have to be the future. How could that change?
Carl Benedikt Frey: That’s right. If you look at growth since the First Industrial Revolution up until quite recently, it’s actually centered around manufacturing goods. Manufacturing goods look quite similar around the world. So if you produce something in China or in the U.S. or in Germany, it doesn’t really matter that much. But when it comes to services, obviously language, and to some degree also culture, matters.
But what we’re seeing as a more immediate consequence of AI, I think, is that it’s alleviating some of those language barriers that previously existed. The service trade in particular is predominantly between English-speaking countries like the United States, India, Bangladesh, [and] the United Kingdom.
AI is already reducing the demand for both translators and language skills, and obviously, now with more recent developments in real-time translation, I think that trend is going to be very much exacerbated. And the good news is that it makes service-led growth possible for a wider set of countries.
Sam Ransbotham: Thanks for taking the time to talk. It was a fascinating keynote and I’m looking forward to reading more about what you’re working on in the future. Thanks.
Carl Benedikt Frey: Thank you for having me, Sam. Great pleasure.
Sam Ransbotham: Our second guest is Karin Kimbrough. She’s the chief economist at LinkedIn. Karin was also speaking on one of the industry panels at the Georgetown/World Bank Jobs in the Age of AI event, and we’re thrilled to be talking with her. Thanks for joining me.
Karin Kimbrough: Thanks for having me.
Sam Ransbotham: LinkedIn is fascinating. You have an amazing insight into a lot that’s going on. Tell us what’s happening.
Karin Kimbrough: It is an amazing place to work, I have to say. As you probably know, LinkedIn is this global platform, which means we have over a billion members who share all of their data with us around their profile, where they went to school, what skills they have, what jobs they’ve had.
All of that helps us piece together this incredible mosaic of career ladders that I think a lot of us don’t have full data on. That’s what makes it so fun. What we’re seeing right now at LinkedIn is a lot around the way employer behavior is shifting. Hiring [is] slowing down globally after that big surge post-pandemic. And we’re seeing a lot of AI — the AI wave — starting to infiltrate the world of work.
Sam Ransbotham: What’s interesting is you have insight into [what] we’ve never had as a society before. If I think back, this is the equivalent of combing through a gazillion classified ads and categorizing them and tagging them. I’m not minimizing what you do, but you’ve got that right at your fingertips really much easier than ever before.
Karin Kimbrough: There’s a lot of that categorizing and tagging and, if you will, almost searching for a needle in a haystack. What we’re doing is trying to look for those green shoots of where AI is starting to show up in the labor force. Part of it is kind of hunting and putting out markers and saying, “Well, why don’t we just search for all the jobs that are called AI-something and see how those are growing? And on the flip side, why don’t we see what jobs maybe aren’t growing, or why don’t we see what skills are more in demand?”
So we’re kind of hunting across this vast lake of data to try to pick up those green shoots, and we’re starting to see some. The tagline is we’re starting to see some evidence of AI permeating the world of work, but I would say it’s still early days to determine where we’re going to be 10 years from now.
Sam Ransbotham: [There are] six things that I want to bother you about there. The first one is, you quickly draw the distinction between jobs and tasks and skills, and I think [there’s], again, something really fascinating about the granularity of the data you have. Tell us about some of those green shoots. What are the green shoots that you’re seeing?
Karin Kimbrough: Let’s break it up into two sides. One side is what [we’ll call] job seekers. Even if you’re working, you might still be a job seeker. What are job seekers doing to change their own behavior? And the other side is what are employers doing? If I start with the individual members on our platform, we can see them starting to add, at a really rapid rate, new AI skills to their profile. Now, you could say, “Do they really have these skills? How do I know?” And that’s a different discussion.
Sam Ransbotham: That’s a different problem.
Karin Kimbrough: It’s a valid point. We do a lot of this. We can also see people doing learning. For example, they’ll come on LinkedIn, and they will start to take courses. They are investing in their own learning. We’ve seen a five times increase in just the past year of people investing in LinkedIn Learning courses around AI.
There were a lot of folks who are investing in what I would call maybe not their “AI expertise” because you might invest in your AI expertise if you want to, say, build a large language model, but you might just want to invest in your “AI literacy.” And that might be, say, an economist or a professor wanting to say, “Well, I’m going to do a little bit more, be more familiar, be more adept at using ChatGPT or Copilot or any number of the different kinds of programs out there that help you with generative AI.” So we’re seeing a huge increase in people learning those skills. It’s not just the techs. It’s also the non-tech folks. And that’s really interesting.
The other side of this is how employers are changing. We’ll come back to that maybe. But we’re seeing lots of new titles that are AI-this, AI-that. There are lots of new roles. There are also roles that have always existed, but now people are just doing different things inside that role. And that’s the element of tasks. If you think of a job as a collection of tasks that require skills, what we think and what we’re seeing is people are rotating the kinds of tasks they do within that same job. A classic example is a marketing manager.
What you’re seeing is people who aren’t necessarily in what you’d consider technical jobs employing a lot of these new technologies, like generative AI, to become more productive and [rotating] away from tasks that are obviously, as we all know, routine. [Those tasks] might be less challenging and, frankly, there’s less value add for them as humans. I think that’s where, if I could add, the most interesting thing that we’re seeing — along with this huge rise in what I would say is people adding AI skills to their profile and employers looking for AI skills — is also a demand by employers for the human skill.
I’ll [also] say that one of the things that we’ve looked at is, what kinds of roles do we think have a high proportion of tasks that might be disrupted by generative AI — if you’re doing a task where generative AI could do two-thirds of the task that you do. Let’s say, for example, you’re transcribing this by hand, this conversation we’re having. You can imagine, is anyone going to pay you to do that? Unless it’s a very elite level of transcription that you’re doing with some nuance of the human element, [the answer is] no one is going to pay you to do that. You will be disrupted from that task. The question is, will there be other tasks that you do in your role that are more important? And for many jobs, people can rotate toward those tasks, but for some jobs, there just isn’t anything left to rotate toward. And those are disrupted jobs, and those do exist.
Sam Ransbotham: What’s interesting if I think about the data you have is you have an ability to see that in a way that we have not. I can complain that you can’t see the hidden uses of AI here, but you can see a lot more than we ever could before. Let’s focus on the fact that we can see a lot of these.
Karin Kimbrough: You could imagine there are disproportionate effects on certain groups, on certain occupations, and maybe — even in the U.S. — certain genders. That’s something that we have to take into account.
Sam Ransbotham: You can break that down pretty fine because you can get a lot more granular than that. I think that was just an example for our conversation, but you’ve got the ability to go a lot more granular.
Karin Kimbrough: We can. I mean, I can give you a number: One-third of the women on our platform are sitting in what I’d call the disrupted bucket, and that’s compared to about a quarter of the men. So women are more likely to be sitting in the disrupted bucket. But they’re not the only ones. Men are there, too.
And then an interesting piece of research that we’re looking at is, what’s the likelihood that you might move out of, let’s say, a role that we would call disrupted at LinkedIn, and move toward something that’s either insulated or augmented? Again, you want to be in the augmented bucket. That’s a really nice place to be. That means I’m using this technology but also leveraging it, and I’m more productive. I’m doing the more fun stuff. What we think we’re finding — it’s early days — is that if you have higher educational attainment, so maybe you have a higher degree, you might be a little more likely to be able to move into that augmented bucket from disrupted. If you have less educational attainment, you might be more likely to move into the insulated bucket.
The insulated bucket is jobs where we don’t think generative AI is really going to eat your lunch right away. Maybe you are a locksmith. Maybe you are a physical therapist. It’s interesting. Educational attainment makes a difference as much as what job you’re starting from and what industry you’re in. Some industries are evolving more quickly than others.
Sam Ransbotham: This has been fun to learn about so many things. Thanks for taking the time to talk.
Karin Kimbrough: Thank you. This was a lot of fun.
Sam Ransbotham: Once the microphones turn off, I’m going to ask you a bunch of detailed questions about what I personally need to do — something that may not be publicly shareable. I’m hoping to get the secrets from you. Thank you.
Karin Kimbrough: Thank you.
Sam Ransbotham: It was great to talk with Carl and with Karin. They were speakers at the event just a minute ago, but now I’m sitting here with Jonathan Timmis. He’s a senior economist at the World Bank who organizes the event. Thanks for putting it on, and thanks for letting me steal some time with a couple of the guests.
To close out this episode, could you share a few takeaways from this year’s event? What do you hope that attendees learn from it?
Jonathan Timmis: I think there were probably four main takeaways from the event. Firstly, I’d say AI is a general-purpose technology, meaning it has the potential to affect a large number of jobs. We heard that 80% of U.S. jobs, for instance, have at least some tasks that are likely to be affected by AI. We heard several industry speakers talk about how AI is helping a variety of workers become more productive in customer service tasks, coding product recommendations, things like this.
But what is actually going to happen to jobs? This is always the really difficult question. I think one thing we can draw from all the evidence we’ve heard today was [that there are] always winners and losers from technology. With previous technologies, like robots or computers, they really benefited the most skilled, often at the expense of those less well-off.
But the evidence so far suggests AI might be a bit different. It seems to benefit lower-ability workers more. For instance, AI translation can reduce the need for foreign language skills, which many low-skilled workers lack. People with relatively basic coding skills can now code really well using AI copilots. So low-skilled people may be able to do new types of work, which sounds like good news, especially for workers in developing countries.
A third point is that AI is just a tool. You really need to know how to work with it to get the gains. This links actually to our first AI in Action conference, where we highlighted that as AI automates prediction-type tasks, things like judgment and knowing what to do with these predictions become really important.
The challenge, of course, is that not all workers are very comfortable using GPTs or copilots, and not all firms [small and medium enterprises] or those in developing countries can afford to give the training that seems to be really important.
The final takeaway is, I would say, about regulation and how this can influence the direction of AI, and where we want AI to go. One of my papers with Tim actually looks at what happens when the U.K. introduces a tax incentive for IT and machinery investments, but cloud services expenses were ineligible for the incentive.
What happened was firms started buying more of their own servers and their own IT rather than buying cloud services. [This] actually slowed down not only the diffusion of cloud in the U.K. but the use of AI, which relies on the cloud, and it slowed down AI use in the U.K. by about one year in total.
In addition, AI has been linked to several risks disinformation. You’ve seen news articles about deepfakes and election interference. And we heard today about a lot of different approaches around the world to try and balance these very real risks against the risks of also not stifling AI development. So I think regulation needs to think broadly, not just about AI models and data but to these other things like tax codes as well.
Sam Ransbotham: Gosh, well, thanks again; I think that was a great summary. Jon and Tim have run this AI in Action series at either the World Bank or at Georgetown for the past few years, but fortunately, they record each event. Our listeners can join these sessions and listen to prior events on demand. In particular, Shervin [Khodabandeh, cohost of Me, Myself, and I] and I were involved in a panel last year on AI in retail and manufacturing, so feel free to check that out. We’ll link these videos in our show notes, and we’ll give you a heads-up when registration opens for the next event in the AI in Action series. Thanks for joining us today.
Allison Ryder: Thanks for listening to Me, Myself, and AI. We believe, like you, that the conversation about AI implementation doesn’t start and stop with this podcast. That’s why we’ve created a group on LinkedIn specifically for listeners like you. It’s called AI for Leaders, and if you join us, you can chat with show creators and hosts, ask your own questions, share your insights, and gain access to valuable resources about AI implementation from MIT SMR and BCG. You can access it by visiting mitsmr.com/AIforLeaders. We’ll put that link in the show notes, and we hope to see you there.