Bussiness
AI Briefing: Inside Accenture and Nvidia’s plan to scale AI agents for enterprise business
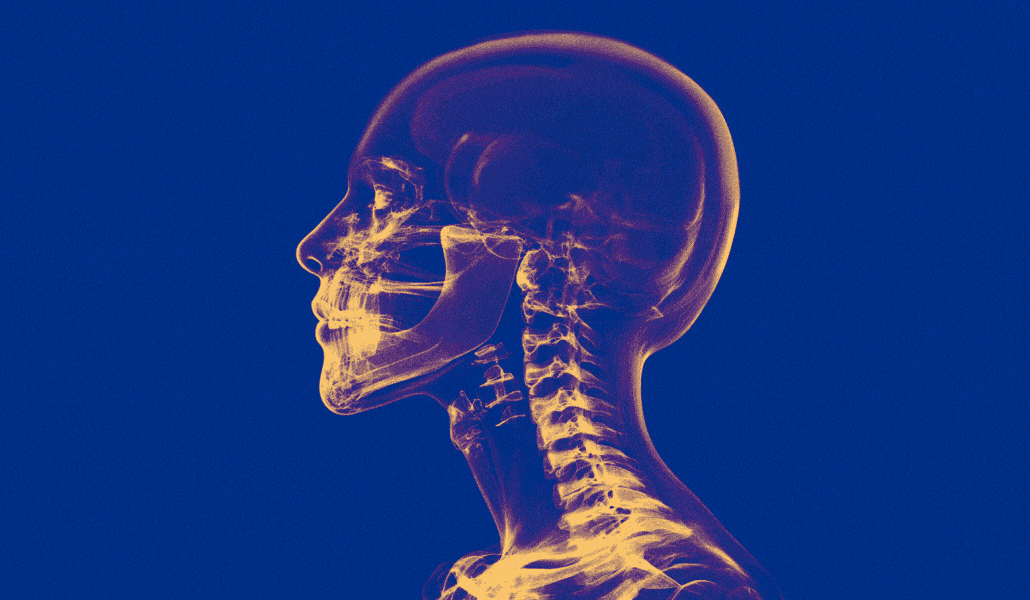
As Accenture and Nvidia seek to accelerate enterprise adoption of AI, their first proving ground has been closer to home: the consulting giant’s own marketing department.
Last month, the companies announced a new Accenture division called Accenture Nvidia Business Group, which entails plans to train more than 30,000 people using Nvidia’s full technology stack: Nvidia AI Foundry for custom AI model creation, AI Enterprise for deploying AI solutions, and Omniverse for 3D simulation and collaboration. The efforts — part of an expanded partnership — also includes Nvidia’s “NIM Agent Blueprint” to help companies more easily develop enterprise-grade AI agents to learn, adapt, and automate complex tasks.
To show how the AI platform works in practice, Accenture’s marketing team wanted to reinvent how the department functions using AI agents, said Accenture CMO Jill Kramer. The first step was seeing which parts of the process might benefit most from agents — including tasks that required the most brainpower or that were the most repetitive.
In a recent interview with Digiday, Kramer explained the end result: More than a dozen agents that have helped cut the total steps in a marketing project from an average of 135 steps to 85. Some of the agents help with marketing research, data analysis, and scheduling social media. Others help search internal documents, draft strategy, and identify how to fund projects based on other parts of a budget.
“Somebody described it to me as thinking about you’re running this track, but you keep having to make a pit stop,” Kramer said. “Research team, pull that data. Analytics team, pull those reports…Depending on the busy-ness of those other teams, you’d get something back in a day, a week, a month, then you’d have to integrate it. Now, [agents] bring it all back to you and they help you integrate it.”
Accenture and Nvidia are just two of the many companies heavily invested in developing, developing and scaling AI agents and copilots. Others in recent months include Snowflake, Salesforce and Microsoft. Last week, Microsoft debuted a new Azure AI Foundry — previously branded as the Azure AI Studio — which came as Microsoft prepares to debut its much anticipated AI Copilot Studio.
Development of enterprise-grade agents has been a part of the ongoing race to embrace generative AI, but driving widespread adoption has been slower than some would like. According to a newly released report from Deloitte, the number of companies launching AI agents will increase to 25% in 2025 and jump to 50% by 2027. Meanwhile, just 30% of AI pilots reach full production. However, analysts expect AI agents to stick around.
“Generative AI and ChatGPT have shown there is consumer appetite, and big tech companies are responding in kind,” said Forrester senior analyst Stephanie Liu. “That said, as with all emerging tech, there is a lot of noise and hype around AI agents, and companies need to do due diligence on identifying what their use case is and what capabilities they realistically need.”
At first, Accenture had tried to give AI tools to as many people at the same time, but Kramer said it created anxiety among those not using them, while the actual use cases were limited. Even after the AI agents were deployed, Accenture had to foster a new mindset to help staffers rethink marketing rather than retrofitting tools into old operating models.
“This is any group anywhere: If you ask them to work in a fundamentally different way, there’s an initial organ rejection,” Kramer said. “The most important part is that ability — we changed our entire operating model. We changed the way our processes flow to make it so that organ rejection wouldn’t happen.”
Rather than make tools for easier tasks like translation, Accenture chief AI officer Lan Guan said the company tackled harder marketing problems. That meant making AI agents for casual inference, strategic planning and pricing optimization. That also requires using powerful computing provided by Nvidia along with the latest large language models like Meta’s Llama 3.
“They want that holistic view, what I call the connected data, so they can actually understand the root causes,” Guan said. “Almost like human detectives when we are putting together those puzzle pieces. With 1,500 puzzle pieces, you do not want to miss a single piece.”
Although Accenture is starting off with marketing, the platform can be operationalized to other parts of business, said Justin Boitano, Nvidia’s vp of enterprise AI. That’s because it was built to consider how teams work together and to embody various roles and personas. Although the early users in Accenture were quickly impressed with early tasks for LLMs, he said enterprise applications take longer to create and improve.
“People realize we’ve got to treat these not just as AI models that magically do everything, but to treat them like employees that we onboard into our company,” said Boitano. “We basically prompt them to do the right thing for a certain business function, we guardrail them to make sure they do a specific job, we evaluate them in doing that job. There’s a process there.”
Adapting general-purpose AI models to understand an enterprise’s specific language, vocabulary, and jargon still remain challenging. Others include skill gaps, performance gaps, rising costs, and regulatory risks. However, Gartner analyst Nicole Greene said AI has proven to support the ability to optimize workflows and create more personalized content. It’ll just take time to develop AI models that have enough agency to act autonomously.
“Marketers should watch out for ‘AI Agent Washing,’ where vendors are announcing AI agents, but few of them will live up to that name,” Greene said. “Because AI agents are designed to act autonomously and proactively in an environment, and often learn and adapt as they operate in their target environment, they pose dramatic risks. Organizations that do not consistently manage AI risks are far more likely to experience adverse outcomes.”
Prompts and Products — AI news and announcements
- Cognitiv and Index Exchange are the latest companies to partner on another way to use deep learning algorithms and large language models for programmatic media.
- With companies like Perplexity and Amazon adding AI tools for e-commerce, there’s growing interest in improving personalization and transparency for search engines powered by LLMs.
- Perplexity announced a new Perplexity Shopping platform to help people find and buy products using generative AI search.
- Pratik Thakar, Coca-Cola’s VP and global head of generative AI, spoke with Digiday about the brand’s AI-generated holiday ad.
- French AI startup Mistral added a new way for its ChatGPT rival “Le Chat” to search the internet and create high-quality images.
- The Content Authenticity Initiative said it now has 4,000 members around around the world from media companies, tech firms, camera makers and smartphone manufacturers.
- Snowflake announced plans to acquire the startup Datavolo to offer multimodal data pipelines. It also has a new deal with Anthropic to bring the latter’s AI models to Snowflake’s data cloud.
- Microsoft announced more ways for its Copilot users to run repetitive tasks for Microsoft 365 apps.
- Amazon expanded use for its AWS AP studio that uses natural language to build and modify apps.
- Pokemon Go’s parent company is reportedly using data from users to train a real-world AI model.
- The Atlantic built a new database that shows all the ways AI models are using Hollywood content.
Thoughts from Humans
People often say generative AI is now easy and accessible to just plug in and use, but Accenture chief AI officer Lan Guan said that’s unrealistic for enterprise organizations. She mentioned one client wanted to use generative AI in their contact center by feeding their chatbot standard operating procedures (SOPs). However, they discovered they had 37 different versions of SOPs, which undermines the idea of a single source of truth.
“What we are seeing here within enterprise organizations is a lot of what we call enterprise messiness,” Guan said. “Data is messy and integration legacy tech is all over the place.”