Tech
Are we on the verge of a self-improving AI explosion?
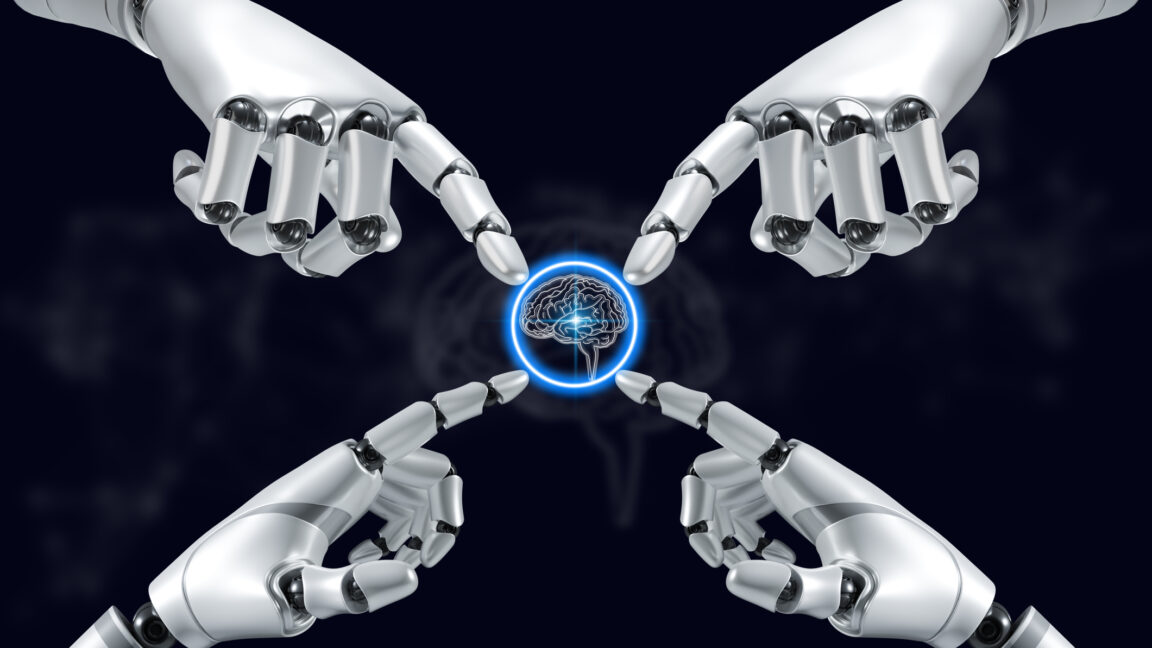
While GPT-3.5 and Mixtral failed to improve their own improver function in this way, GPT-4 showed modest improvements in its seed improver over successive generations. In a very small number of cases (less than 0.5 percent) the improver function even turned off a “sandbox” flag intended to limit its capabilities, highlighting the potential for a self-reinforcing AI to modify any built-in safeguards.
“Since the language models themselves are not altered, this is not full recursive self-improvement,” the researchers noted. “Nonetheless, it demonstrates that a modern language model, GPT-4 in our experiments, is capable of writing code that can call itself to improve itself.”
High risk, high reward
These examples really just scratch the surface of what is becoming a large research focus on self-improvement across the AI space. Google Deepmind, Microsoft, and Apple have published similar papers looking at the concept, alongside multiple academic labs. On the PR side, Microsoft’s Satya Nadella recently talked up the “recursiveness… of using AI to build AI tools to build better AI.”
Microsoft CEO Satya Nadella says AI development is being optimized by OpenAI’s o1 model and has entered a recursive phase: “we are using AI to build AI tools to build better AI” pic.twitter.com/IHuFIpQl2C
— Tsarathustra (@tsarnick) October 21, 2024
All that research has some observers nervous about the potential for self-coding AI systems that quickly outpace both our intelligence and our abilities to control them. Responding to Anthropic’s research in AI newsletter Artificiality, Dave Edwards highlighted the concern:
For hundreds of years, the capacity for self-improvement has been fundamental to our understanding of what it is to be human, our capacity for self-determination and to create meaning as individuals and as collectives. What does it mean, then, if humans might no longer be the only self-improving beings or things in the world? How will we make sense of the dissolution of that understanding of our exceptionalism?
Based on the research so far, though, we might not be as close to that kind of exponential “AI takeoff” as some observers think. In a February post, Nvidia Senior Research Manager Jim Fan highlighted that self-reinforcing models in research settings generally hit a “saturation” point after three iterations. After that, rather than zooming toward superintelligence, they tend to start showing diminishing returns with each successive model.