Tech
Challenges in Training Generative AI Models
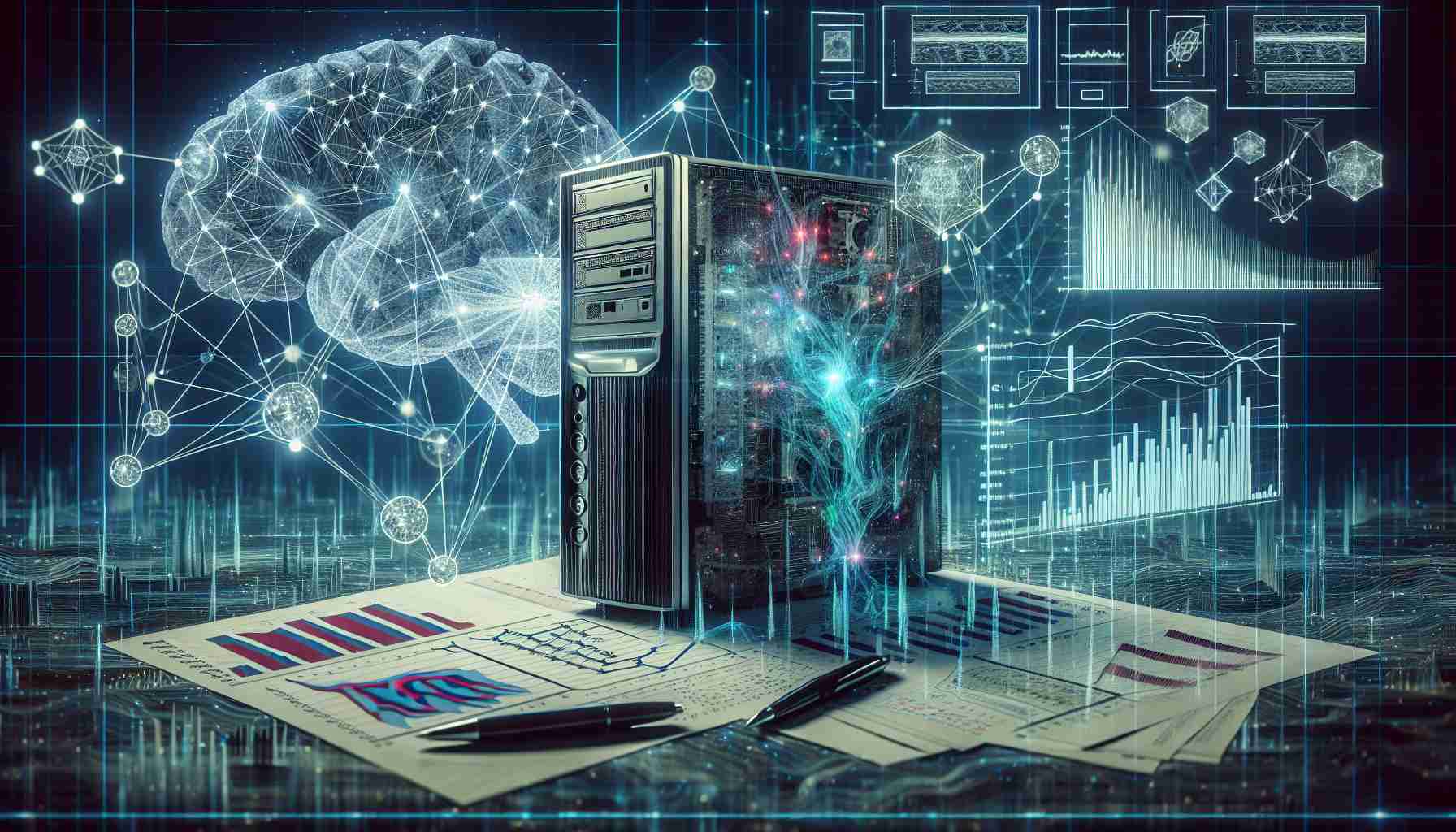
New Unlearning Techniques Present Complex Challenges for AI Models
Researchers have discovered that the latest unlearning techniques are causing issues for advanced AI models such as GPT-4o and Llama 3.1 405B, as reported by a collaborative study among institutions like the University of Washington, Princeton, the University of Chicago, USC, and Google. The study indicates that current unlearning methods are not yet effective in erasing specific data without damaging the model’s overall functionality.
The Learning Process of AI Models
Generative AI models function based on patterns they have learned from vast amounts of data. For example, when fed email data closing with “Looking forward…”, the autocomplete feature predicts the phrase “… to hearing back.” These models lack intent and solely rely on statistical analysis to suggest responses.
Copyright Conundrum and the Rise of Unlearning Techniques
Unauthorized data scraping by AI model developers from public sources has led to copyright disputes with individuals and organizations like authors, publishers, and record labels. Unlearning techniques have attracted significant attention in response to this issue, with Google initiating competitions to encourage the development of efficient methods for model correction.
Challenges in Implementing Unlearning
Unlearning strategies attempt to guide models away from specific data patterns to enhance data privacy. However, affecting the model’s predictions can result in reduced performance when answering questions. Shi and her team introduced the Machine Unlearning Six-way Evaluation (MUSE) benchmark to analyze the impact of unlearning on the model’s retention of information.
Future Prospects and Continuing Research
The study underlines the complexity of unlearning techniques and emphasizes the necessity for further exploration in this field. While unlearning could hold promise for future AI data management, current challenges suggest that more research is essential to overcome the limitations posed by existing methods.
Enhancing Generative AI Models: Overcoming Challenges and Unveiling New Insights
In delving deeper into the realm of generative AI models, alongside the intricate landscape of unlearning techniques, an array of crucial nuances and complexities emerges that demand attention. As we explore the dynamic interplay between cutting-edge AI advancements and the evolving challenges they bring, several pivotal questions come to the fore, each beckoning for insightful answers.
Pioneering Questions and Insightful Answers
1. **How do different training strategies impact the utility and adaptability of generative AI models?**
– While traditional training methods are vital, newer approaches like continual learning and self-supervised learning offer fresh insights into enhancing model performance and adaptability.
2. **What role does data diversity play in shaping the capabilities of generative AI models?**
– Diverse datasets not only enrich model understanding but also pose challenges related to bias, generalization, and ethical considerations within AI applications.
3. **How can interpretability and explainability be integrated into generative AI models without compromising performance?**
– Balancing explainability with the complexity of advanced AI models remains a significant challenge, necessitating innovative solutions that offer transparency without sacrificing efficiency.
Key Challenges and Controversies
One of the central dilemmas in the domain of generative AI models lies in navigating the delicate balance between data privacy and model performance. While unlearning techniques present a tantalizing proposition for enhancing privacy safeguards, their implementation raises critical challenges.
**Unintended Effects:** Implementing unlearning methods to enhance privacy may inadvertently disrupt the model’s predictive accuracy and responsiveness, underscoring the need for nuanced strategies that minimize adverse impacts.
**Ethical Implications:** The use of unlearning techniques raises ethical dilemmas surrounding data ownership, consent, and the responsible management of sensitive information, necessitating a robust ethical framework to guide AI model development and deployment.
**Technical Limitations:** The complexity inherent in unlearning processes introduces technical hurdles related to scalability, efficiency, and the preservation of past learning, highlighting the need for innovative solutions that mitigate these limitations.
Advantages and Disadvantages
**Advantages:**
– Enhanced Data Privacy: Unlearning techniques offer a pathway to fortify data privacy measures within AI models, fostering trust and compliance with regulatory standards.
– Adaptive Learning: By enabling models to adapt and evolve based on changing data dynamics, unlearning approaches enhance model flexibility and resilience.
**Disadvantages:**
– Performance Trade-Offs: The trade-off between privacy protection and model performance poses a significant drawback, necessitating careful calibration to strike a balance.
– Implementation Complexity: Integrating unlearning mechanisms into existing AI infrastructures demands specialized expertise and resources, potentially hindering widespread adoption.
As we navigate the intricate landscape of generative AI models and grapple with the multifaceted challenges of unlearning techniques, a myriad of opportunities for innovation and advancement beckon. By critically examining these complexities, engaging with ethical considerations, and driving forward collaborative research efforts, we pave the way for a future where generative AI models can thrive responsibly and ethically.
For further exploration on the latest advancements in AI research and technologies, visit OpenAI.