Bussiness
Driving Business Growth with GreenTomato’s Data and Machine Learning Strategy on Generative AI | Amazon Web Services
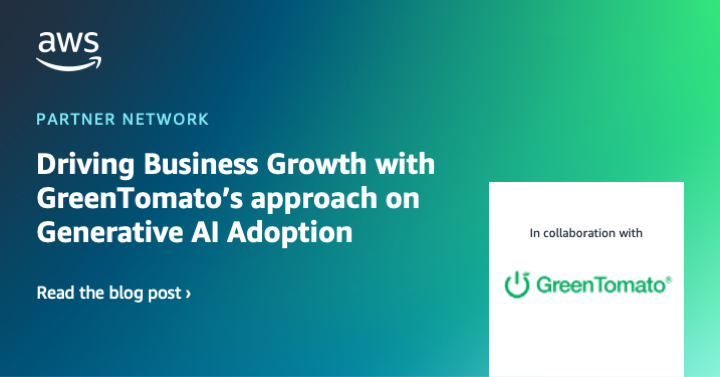
By Scott Lam, Senior Technical Manager – Green Tomato
By Anita Wong, Technology Architect – Green Tomato
By Cow Cheng, Senior Machine Learning Engineer – Green Tomato
By Lok Yeung, Associate Partner Solutions Architect – AWS
Green Tomato Limited |
![]() |
In today’s digital transformation era, generative AI is revolutionizing how organizations engage with data and extract insights as the outputs. With the need of enhancing outputs from data with accuracy and context, organizations are leveraging their extensive datasets with the adoption of Retrieval-Augmented Generation (RAG). To drive our customers on the adoption of RAG, GreenTomato follows a comprehensive approach for generative AI adoption from data strategy to RAG application.
GreenTomato adopts the above approach to drive successful innovations in key industries, including but not limited to enhancing customer experience in shopping malls, improving customer services and customer engagement in retail, and enabling precise risk assessment in financial services. These applications empower businesses to make informed decisions and offer tailored user experiences.
GreenTomato’s Approach on generative AI adoption
At GreenTomato, we integrate generative AI and RAG into business operations through a comprehensive data strategy that focuses on accuracy and efficiency. Our approach involves the following:
- Data Strategy: Building a solid data foundation through meticulous data collection, cleaning, and preprocessing to provide high-quality inputs for AI models.
- ETL Pipeline: Creating efficient extract, transform, load (ETL) pipelines to integrate data from data sources, making sure that it is up-to-date and available for analysis and AI training.
- Retrieval-Augmented Generation (RAG): Enhancing generative models with real-time data retrieval to improve the relevance of generated content.
- Optical Character Recognition (OCR): Utilizing OCR to convert documents like scanned papers and PDFs into editable, searchable formats, improving data accessibility.
- Seamless Integrations: Enabling integrations with existing systems to build an interoperable AI landscape for maximum efficiency.
This approach helps customers to leverage advanced AI capabilities, improve decision-making, enhance user experiences, and drive growth and competitive advantage.
Data Strategy: A Robust Foundation
Understanding that the quality of generated content depends on underlying data, GreenTomato has developed a specialized data strategy that includes meticulous data collection, cleaning, and preprocessing.
Data Collection: GreenTomato focuses on collecting data from diverse, reliable sources to build comprehensive datasets. This diversity is crucial for training robust RAG models capable of handling inputs and queries. For instance, in the financial sector, data collection involves aggregating transaction records, customer profiles, industry data, and compliance documents. By using AWS services like Amazon Kinesis for real-time data streaming and Amazon Relational Database Service (RDS) for database management, financial institutions can process large volumes of data efficiently, enabling personalized financial advice, fraud detection, and better risk management.
Data Cleansing: After collection, the next crucial step is data cleaning. This involves removing inaccurate, outdated, or irrelevant information, providing trustworthy outputs from the generative models. In finance, data cleaning includes validating transactions, updating customer profiles, and making sure that industry data is current. AWS Glue can automate tasks like deduplication, normalization, and anomaly detection, maintaining accurate datasets that enhance the reliability of AI outputs.
Data Preprocessing: The preprocessing stage prepares cleaned data for RAG models using techniques like tokenization (breaking text into smaller, manageable units) and semantic tagging (assigning meaningful labels to tokens). This improves the model’s understanding of context and word significance, providing more accurate and relevant outputs. Amazon Comprehend can handle tokenization and semantic tagging, facilitating nuanced data handling.
Building an ETL Pipeline with AWS Services
GreenTomato’s ETL pipeline showcases its innovative use of AWS services, with the high-level architecture summarized in Figure 1. With Amazon Kinesis, we efficiently stream real-time data for timely extraction and processing. Data is securely stored in Amazon S3, while Amazon SageMaker is essential for model training and deployment. This integration streamlines operations and enhances our capacity to manage large-scale data workflows, vital for training advanced RAG models.
Figure 1 ETL workflow diagram with simple OCR example
Enhancing RAG with Advanced Data Annotation
Data annotation is critical for refining RAG models, providing a detailed information map that helps AI understand context and nuances better. For example, NLP models often struggle with understanding context, sentiment, or intent in text due to the nuances of human language.
Annotating text data with labels for sentiment, entities, or intent helps improve the model’s understanding. For instance, sentiment analysis models can be trained on annotated datasets to accurately classify text as positive, negative, or neutral.
GreenTomato employs a dual approach to provide accurate annotations, including manual annotations by domain experts and automated annotations using advanced algorithms. In terms of manual annotations by domain experts, experts provide high-quality, nuanced annotations in addition to automated process in order to promoting a rich and contextually accurate dataset. For automated annotations using advanced algorithms, algorithms handle large datasets and straightforward tasks in order to continuously refining the annotations with efficiency and scale.
Integrating OCR for Unstructured Data
Recognizing the value of unstructured data like images, GreenTomato has developed an Optical Character Recognition (OCR) model tailored for extracting textual data from images. This allows for incorporating diverse data sources, like scanned documents and social media images, into RAG systems, significantly broadening the data scope and enhancing generative capabilities. Figure 2 below shows the simple RAG architecture that in corporate different RAG engines that leveraging different data sources, including Amazon S3, Amazon OpenSearch, and Pinecone vector database.
Figure 2 RAG architecture diagram
Retail Industry Example: A leading e-commerce company implemented GreenTomato’s OCR model to enhance their data strategy. By extracting text from sources like scanned receipts, product labels, and customer-shared images on social media, the company tapped into a vast pool of unstructured data, capturing valuable information that was previously inaccessible. Using AWS Textract, they automated the extraction of text from images, while AWS Comprehend provided semantic tagging and sentiment analysis, streamlining comprehensive and accurate data collection.
Optimization and Future Implications: To optimize the OCR model, GreenTomato employed advanced machine learning techniques and neural networks specializing in pattern recognition and text extraction. Continuous training with diverse datasets further enhances the model’s accuracy and efficiency. The integration of OCR technology into RAG systems opens new avenues for data utilization, such as sentiment analysis from images or contextual advertising based on text found in videos. This makes sure that GreenTomato’s clients remain at the forefront of AI-driven data strategies, continuously enhancing operational capabilities and customer experiences.
Innovating with Partners and Broader Implications
GreenTomatos is committed to pushing the boundaries of what’s possible with AI. We actively seek partnerships with other technology leaders and innovators among industries to explore new applications and tackle complex problems. By combining our expertise in RAG and generative AI with the capabilities of AWS and other partners, we aim to create solutions that are not only technologically advanced, but also strategically aligned with industry needs and future trends.
Conclusion
With AWS technologies, GreenTomato’s comprehensive approach to generative AI and RAG is transforming how businesses leverage their data across industries. Our solutions drive tangible outcomes, from enhancing retail customer experiences to optimizing financial risk assessment.
Green Tomato Limited – AWS Partner Spotlight
Green Tomato, an AWS Partner, empowers businesses in digital transformation to create innovative products and impactful experiences.
Contact GreenTomato | Partner Overview |