Tech
Liquid AI Announces First Generation of Language Liquid Foundation Models
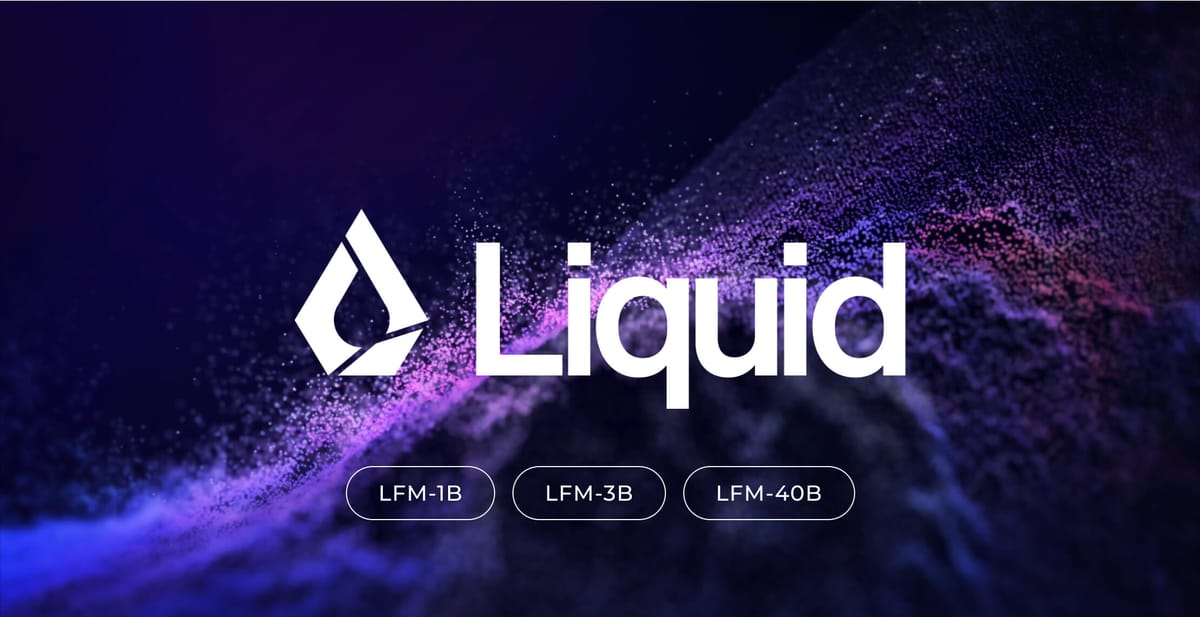
Liquid AI has introduced a new series of language models that promise to reshape the AI landscape. The company’s Liquid Foundation Models (LFMs) achieves state-of-the-art performance while maintaining a smaller memory footprint and more efficient inference compared to traditional transformer-based architectures.
The release includes three models: LFM-1B
, LFM-3B
, and LFM-40B
. Each targets a specific niche in the AI ecosystem, from resource-constrained environments to more complex tasks requiring larger parameter counts.
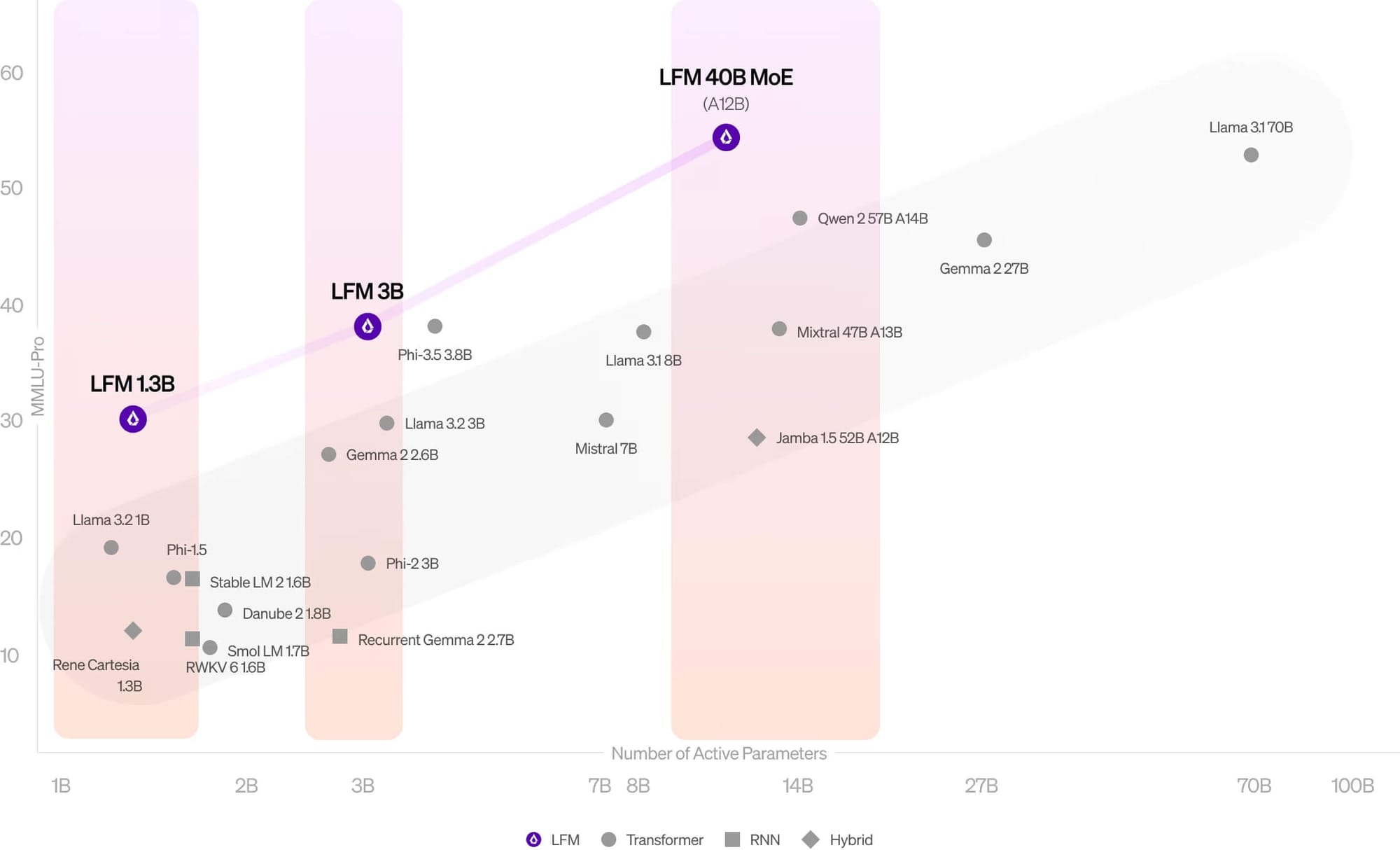
LFM-1B, with 1.3 billion parameters, sets a new benchmark in its size category. It’s the first non-GPT architecture to significantly outperform transformer-based models of similar scale across various public benchmarks.
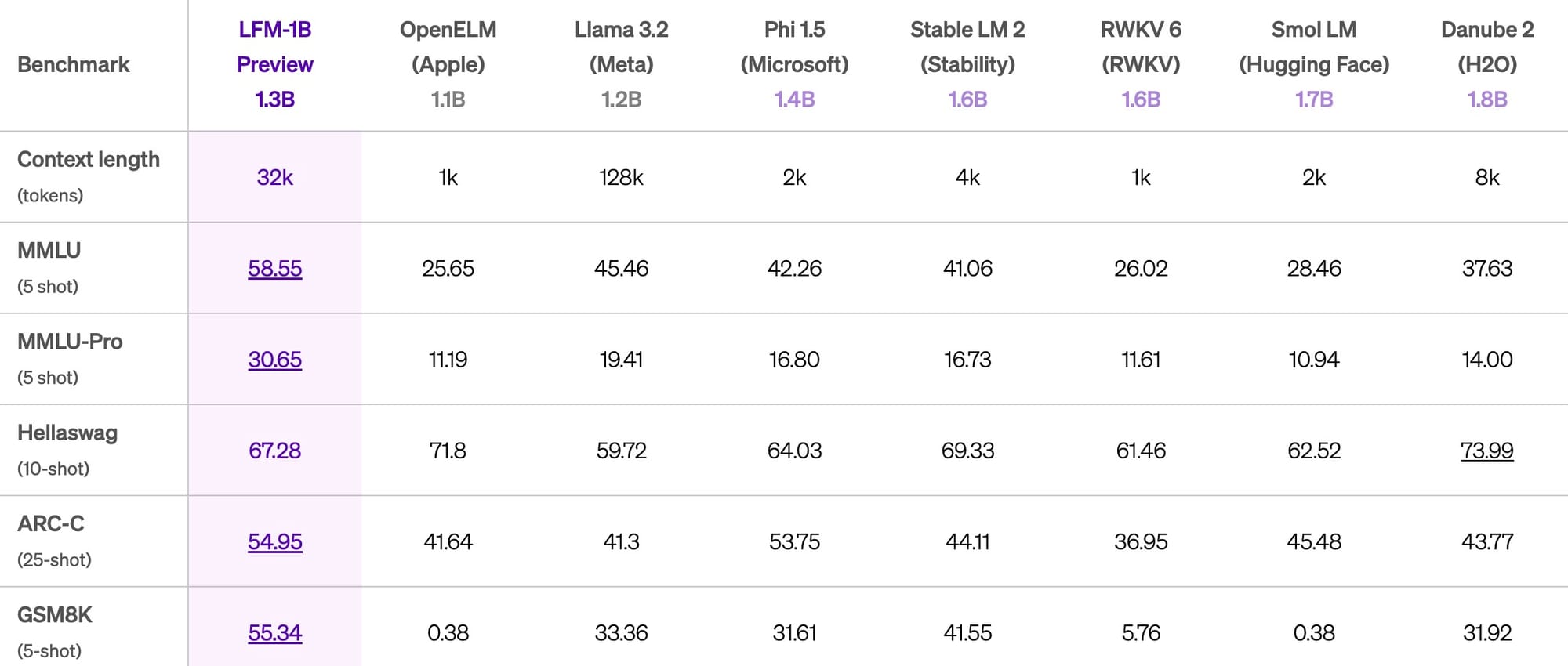
The 3.1 billion parameter LFM-3B is positioned for edge deployment, particularly in mobile applications. Liquid AI reports that it not only outperforms other 3B models but also surpasses some 7B and 13B parameter models. The company claims it matches the performance of Microsoft’s Phi-3.5-mini on multiple benchmarks while being 18.4% smaller.
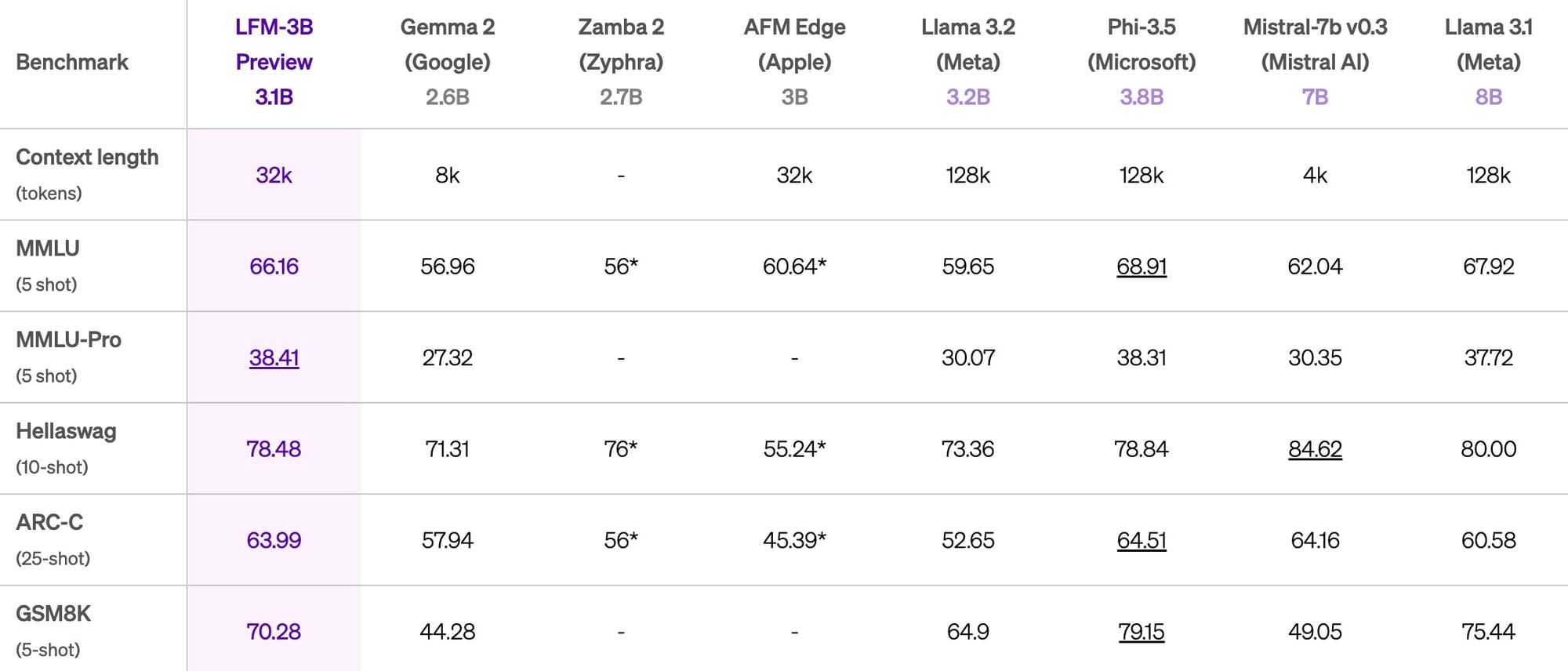
At the high end, LFM-40B employs a Mixture of Experts (MoE) architecture, activating 12 billion parameters during use. Liquid AI states this model delivers performance comparable to larger models while enabling higher throughput on more cost-effective hardware.
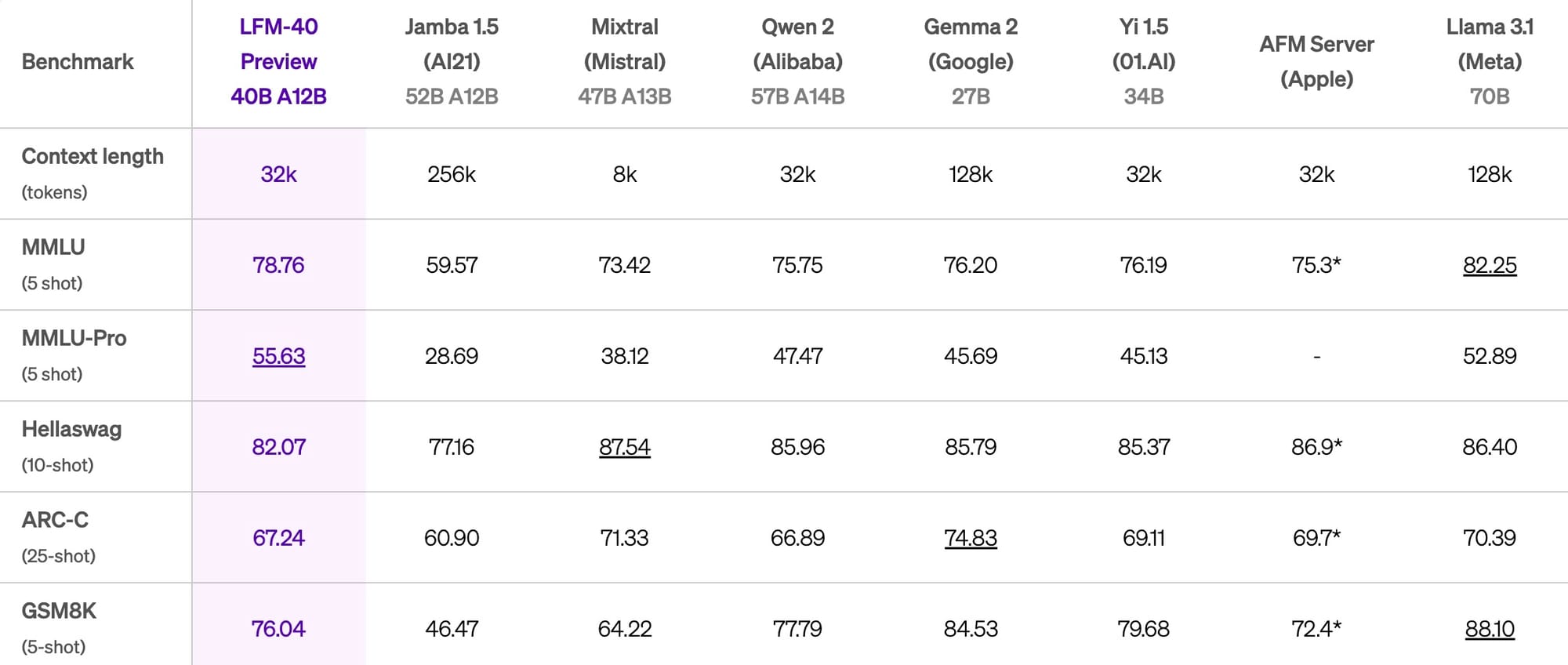
A key differentiator for LFMs is their approach to handling long inputs. Unlike transformer models, where memory usage grows linearly with input length, LFMs maintain a more constant memory footprint. This efficiency allows them to process longer sequences on the same hardware, with the company citing an optimized 32,000 token context length across its models.
Liquid AI’s approach diverges from the prevalent transformer-based architectures, instead building on principles from dynamical systems, signal processing, and numerical linear algebra. The company claims this foundation allows LFMs to leverage decades of theoretical advances in these fields.
Despite these impressive capabilities, Liquid AI acknowledges current limitations. The models struggle with zero-shot code tasks, precise numerical calculations, time-sensitive information, and more.
If you want to give LFMs a try, you have a few options—Liquid Playground, Lambda (via both Chat UI and API), and Perplexity Labs. The company also announced that Cerebras Inference will soon support these models. Additionally, Liquid AI is optimizing the LFM stack for hardware from NVIDIA, AMD, Qualcomm, Cerebras, and Apple, potentially broadening their accessibility across different computing environments.
However, despite the impressive benchmarks and innovative architecture of Liquid AI’s Liquid Foundation Models, it’s important to recognize that this technology is still in its early stages. While the models show great promise on paper, their real-world effectiveness and scalability have yet to be fully tested. As the AI community continues to explore new model architectures, LFMs represent a noteworthy development that certainly could influence future directions. However, only time and practical application will determine their true impact on the broader AI landscape.