Tech
What’s an NPU and Why Is Big Tech Suddenly Obsessed?
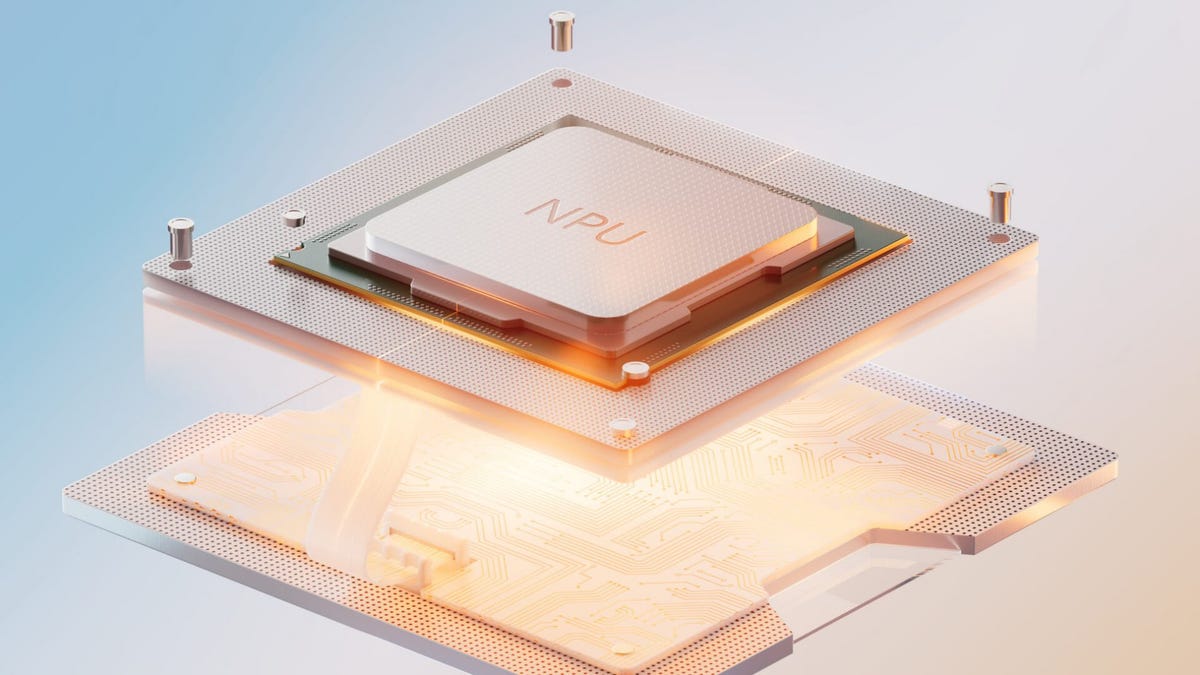
There’s a CPU. There’s a GPU. In the past year, every tech company has been talking about “NPUs.” If you didn’t know the first two, you’re probably flummoxed about the third and why every the tech industry is extolling the benefits of a neural processing unit. As you might have guessed, it’s all due to the ongoing hype cycle around AI. And yet, tech companies have been rather bad at explaining what these NPUs do or why you should care.
Everybody wants a piece of the AI pie. Google said “AI” more than 120 times during this month’s I/O developer conference, where the possibilities of new AI apps and assistants practically enraptured its hosts. During its recent Build conference, Microsoft was all about its new ARM-based Copilot+ PCs using the Qualcomm Snapdragon X Elite and X Plus. Either CPU will still offer an NPU with 45 TOPS. What does that mean? Well, the new PCs should be able to support on-device AI. However, when you think of it, that’s exactly what Microsoft and Intel promised late last year with the so-called “AI PC.”
If you bought a new laptop with an Intel Core Ultra chip this year on the promise of on-device AI, you’re probably none too happy with getting left behind. Microsoft has told Gizmodo that only the Copilot+ PCs will have access to AI-based features like Recall “due to the chips that run them.”
However, there was some contention when well-known leaker Albacore claimed they could run Recall on another ARM64-based PC without relying on the NPU. The new laptops aren’t yet available, but we’ll need to wait and see how much pressure the new AI features put on the neural processors.
But if you’re truly curious about what’s going on with NPUs and why everyone from Apple to Intel to small PC startups are talking about them, we’ve concocted an explainer to get you up to speed.
Explaining the NPU and ‘TOPS’
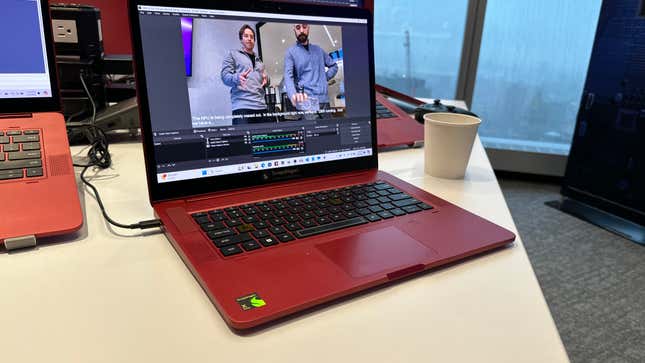
So first, we should offer the people in the background a quick rundown of your regular PC’s computing capabilities. The CPU, or “central processing unit,” is—essentially—the “brain” of the computer processing most of the user’s tasks. The GPU, or “graphics processing unit,” is more specialized for handling tasks requiring large amounts of data, such as rendering a 3D object or playing a video game. GPUs can either be a discrete unit inside the PC, or they can come packed in the CPU itself.
In that way, the NPU is closer to the GPU in terms of its specialized nature, but you won’t find a separate neural processor outside the central or graphics processing unit, at least for now. It’s a type of processor designed to handle the mathematical computations specific to machine learning algorithms. These tasks are processed “in parallel,” meaning it will break up requests into smaller tasks and then process them simultaneously. It’s specifically engineered to handle the intense demands of neural networks without leveraging any of the other systems’ processors.
The standard for judging NPU speed is in TOPS, or “trillions of operations per second.” Currently, it’s the only way big tech companies are comparing their neural processing capability with each other. It’s also an incredibly reductive way to compare processing speeds. CPUs and GPUs offer many different points of comparison, from the numbers and types of cores to general clock speeds or teraflops, and even that doesn’t scratch the surface of the complications involved with chip architecture. Qualcomm explains that TOPS is just a quick and dirty math equation combining the neural processors’ speed and accuracy.
Perhaps one day, we’ll look at NPUs with the same granularity as CPUs or GPUs, but that may only come after we’re over the current AI hype cycle. And even then, none of this delineation of processors is set in stone. There’s also the idea of GPNPUs, which are basically a combo platter of GPU and NPU capabilities. Soon enough, we’ll need to break up the capabilities of smaller AI-capable PCs with larger ones that could handle hundreds or even thousands of TOPS.
NPUs Have Been Around for Several Years on Both Phones and PCs
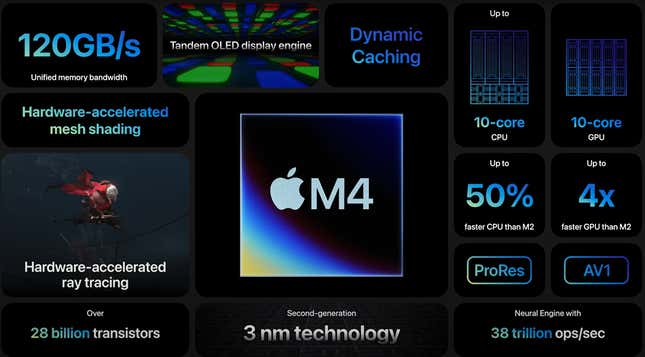
Phones were also using NPUs long before most people or companies cared. Google talked about NPUs and AI capabilities as far back as the Pixel 2. Chinese-centric Huawei and Asus debuted NPUs on phones like 2017’s Mate 10 and the 2018 Zenphone 5. Both companies tried to push the AI capabilities on both devices back then, though customers and reviewers were much more skeptical about their capabilities than today.
Indeed, today’s NPUs are far more powerful than they were six or eight years ago, but if you hadn’t paid attention, the neural capacity of most of these devices would have slipped by you.
Computer chips have already sported neural processors for years before 2023. For instance, Apple’s M-series CPUs, the company’s proprietary ARC-based chips, already supported neural capabilities in 2020. The M1 chip had 11 TOPS, and the M2 and M3 had 15.8 and 19 TOPS, respectively. It’s only with the M4 chip inside the new iPad Pro 2024 that Apple decided it needed to boast about the 38 TOPS speed of its latest neural engine. And what iPad Pro AI applications truly make use of that new capability? Not many, to be honest. Perhaps we’ll see more in a few weeks at WWDC 2024, but we’ll have to wait and see.
The Current Obsession with NPUs Is Part Hardware and Part Hype
The idea behind the NPU is that it should be able to take the burden of running on-device AI off the CPU or GPU, allowing users to run AI programs, whether they’re AI art generators or chatbots, without slowing down their PCs. The problem is we’re all still searching for that one true AI program that can use the increased AI capabilities.
Gizmodo has had conversations with the major chipmakers over the past year, and the one thing we keep hearing is that the hardware makers feel that, for once, they’ve outpaced software demand. For the longest time, it was the opposite. Software makers would push the boundaries of what’s available on consumer-end hardware, forcing the chipmakers to catch up.
But since 2023, we’ve only seen some marginal AI applications capable of running on-device. Most demos of the AI capabilities of Qualcomm’s or Intel’s chips usually involve running the Zoom background blur feature. Lately, we’ve seen companies benchmarking their NPUs with AI music generator model Riffusion in existing applications like Audacity or with live captions on OBS Studio. Sure, you can find some apps running chatbots capable of running on-device, but a less capable, less nuanced LLM doesn’t feel like the giant killer app that will make everybody run out to purchase the latest new smartphone or “AI PC.”
Instead, we’re limited to relatively simple applications with Gemini Nano on Pixel phones, like text and audio summaries. Google’s smallest version of its AI is coming to the Pixel 8 and Pixel 8a. Samsung’s AI features that were once exclusive to the Galaxy S24 have already made their way to older phones and should soon come to the company’s wearables. We haven’t benchmarked the speed of these AI capabilities on older devices, but it does point to how older devices from as far back as 2021 already had plenty of neural processing capacity.
On-device AI is still hampered by the lack of processing power for consumer-end products. Microsoft, OpenAi, and Google need to run major data centers sporting hundreds of advanced AI GPUs from Nvidia, like the H100 (Microsoft and others are reportedly working on their own AI chips), to process some of the more advanced LLMs or chatbots with models like Gemini Advanced or GPT 4o. This is not cheap in terms of either money or resources like power and water, but that’s why so much of the more advanced AI consumers can pay for it is running in the cloud. Having AI run on the device benefits users and the environment. If companies think consumers demand the latest and greatest AI models, the software will continue to outpace what’s possible on a consumer-end device.